In the world of data analysis, handling missing values is a critical aspect that can significantly impact the outcome of your research and findings. IBM SPSS, a powerful statistical software, provides various tools and techniques to manage these missing values effectively. By understanding how to navigate through missing data, analysts can ensure that their analyses are robust and reliable. This article will delve deep into the intricacies of IBM SPSS missing values, offering insights into how to identify, handle, and interpret them in your datasets.
Missing values can arise for numerous reasons, including data collection errors, respondent non-responses, or data entry mistakes. Regardless of the cause, addressing these gaps in your data is crucial for maintaining the integrity of your analysis. IBM SPSS offers researchers multiple strategies for dealing with missing values, including deletion methods, imputation techniques, and the use of specialized algorithms.
By equipping yourself with the knowledge of IBM SPSS missing values, you can enhance the accuracy of your statistical analyses and make informed decisions based on your findings. This guide will explore the various aspects of missing values in SPSS, including how to identify them, the implications of leaving them unaddressed, and the best practices for managing them within your datasets.
What Are Missing Values in IBM SPSS?
Missing values refer to the absence of data points in a dataset. In IBM SPSS, these can occur for several reasons, such as:
- Non-response from survey participants
- Errors during data entry
- Data corruption or loss
Understanding the nature and extent of missing values is critical for statistical analysis, as they can alter the results significantly.
How Can You Identify Missing Values in IBM SPSS?
To effectively manage missing values, you must first identify their presence within your dataset. IBM SPSS provides several methods for this:
- Using Descriptive Statistics: Generate frequency tables to identify missing values.
- Utilizing the Missing Value Analysis Tool: This feature offers a comprehensive overview of missing data patterns.
- Employing Visualizations: Graphical representations, such as histograms or box plots, can highlight gaps in your data.
What Are the Implications of Missing Values in Your Analysis?
Failing to address missing values can lead to biased results and misinterpretations. Some implications include:
- Reduced sample size, leading to less reliable results
- Increased variance in statistical estimates
- Potential loss of significant relationships between variables
It is essential to consider these factors when planning your analysis.
What Techniques Are Available for Handling Missing Values in IBM SPSS?
IBM SPSS offers several strategies for addressing missing values, including:
- Listwise Deletion: Involves excluding any case with missing data from the analysis.
- Pairwise Deletion: Allows for the use of available data points for calculations, rather than excluding entire cases.
- Mean Substitution: Replaces missing values with the mean of the observed values.
- Multiple Imputation: A more sophisticated approach that generates multiple datasets with imputed values.
How to Perform Missing Value Analysis in IBM SPSS?
To conduct a missing value analysis in IBM SPSS, follow these steps:
- Open your dataset in SPSS.
- Navigate to the “Analyze” menu, then select “Missing Value Analysis.”
- Choose the variables you want to analyze.
- Review the summary output to understand the patterns of missing data.
What Are Common Mistakes to Avoid When Dealing with Missing Values?
When handling missing values, it's crucial to avoid common pitfalls, such as:
- Ignoring missing data altogether
- Choosing inappropriate imputation methods
- Failing to report how missing values were handled in your analysis
Awareness of these mistakes can lead to more accurate and reliable research outcomes.
Can You Use IBM SPSS to Visualize Missing Values?
Yes, IBM SPSS provides tools for visualizing missing values, which can enhance your understanding of data patterns. By using graphical representations, you can:
- Identify trends in missing data
- Assess the impact of missing values on your analysis
- Communicate findings effectively to stakeholders
Conclusion: Why Is It Important to Address Missing Values in IBM SPSS?
Addressing missing values in IBM SPSS is essential for ensuring the accuracy and reliability of your statistical analyses. By employing appropriate techniques and remaining vigilant about potential pitfalls, researchers can enhance the quality of their findings. With a proper understanding of IBM SPSS missing values, you can make informed decisions and contribute valuable insights to your field of study.


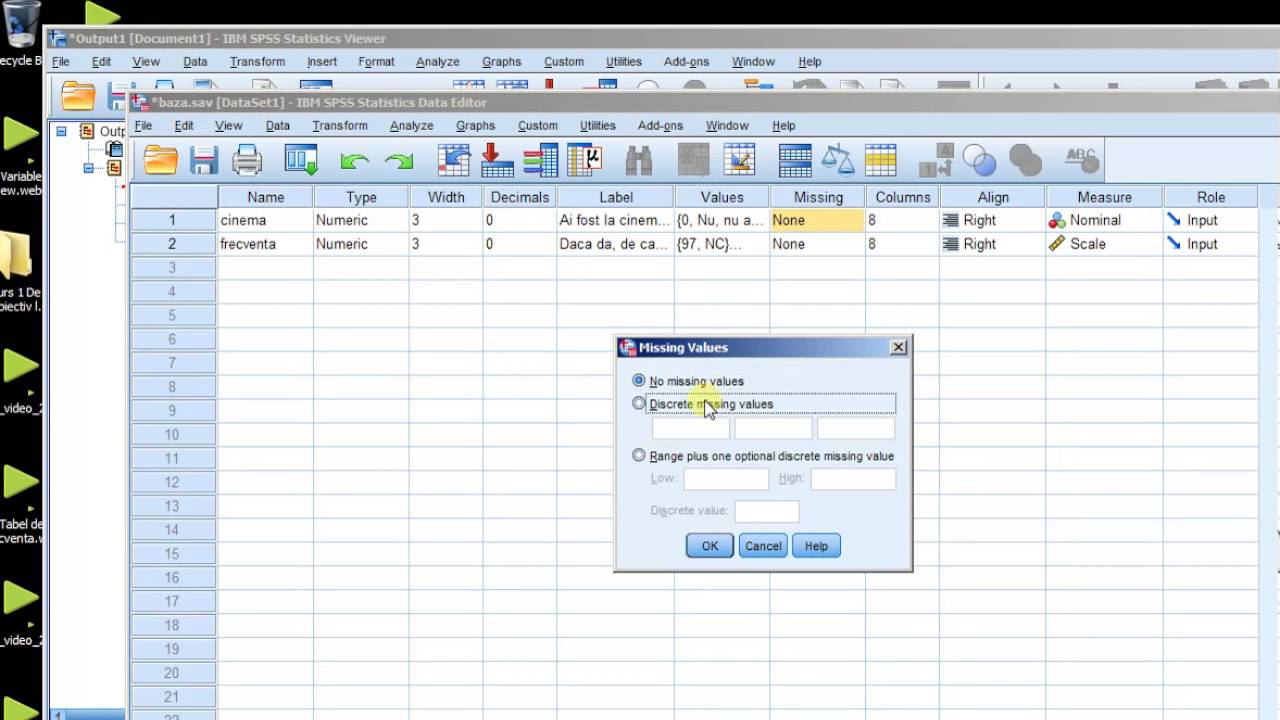